GOOGLE BERT
08 Aug
WHAT IS IT AND SHOULD IT CHANGE YOUR APPROACH TO SEO
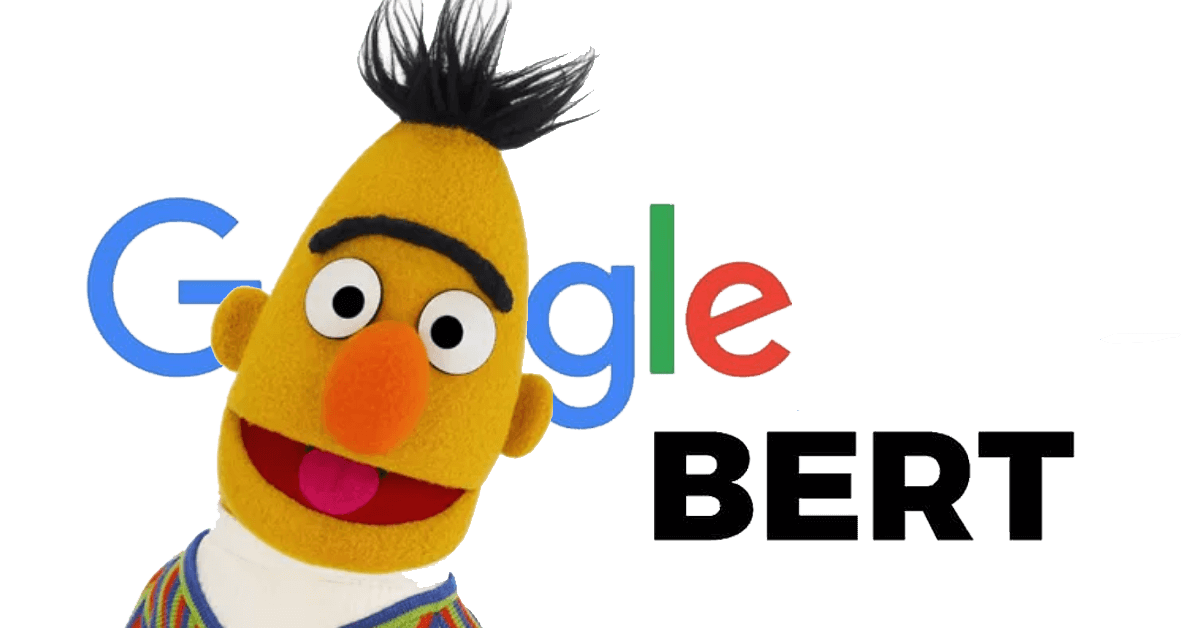
What is Google BERT?
BERT stands for Bidirectional Encoder Representations from Transformers.
While this sounds complex, all we need to know is that it’s Google’s way to better understand the finer details of the natural language that we use.
In fact, before Google introduced BERT as part of its main algorithm, it was released as an open-sourced neural network.
This meant that anyone with the technical know-how could use the code to train their own state-of-the-art question answering system.
Wow, that sounds like a lot of fun – but how does BERT work?
How Does Google BERT work?
In the past, Google hasn’t always been great at understanding complex or conversational searches.
And according to Google, “people often type strings of words that they think we’ll understand but aren’t actually how they’d naturally ask a question”.
This phenomenon is known in Google HQ as “keyword-ese,” and it's something Google wants to avoid.
So, through machine learning and natural language processing, BERT processes the full context of a word from a query. It then reviews the words that come before and after it, rather than just focusing on each word at a time.
Before releasing BERT into the wild, Google did a lot of testing. Testing to see whether the before and after results were improved.
For the keyword “2019 brazil traveler to usa need a visa” the preposition “to” is important.
This shows that the traveler wants to visit USA from Brazil, and not the other way around.
Before BERT, Google didn’t understand this nuance or the full context of the query and would promote results for USA visitors travelling to Brazil, which, of course, isn’t what the searcher expects!
For the keyword “parking on a hill with no curb,” Google would previously be confused by this query. It would put too much emphasis on the keyword “curb” and ignore the word “no.” Again, delivering results which weren’t necessarily what the user expects.
From Keyword Matching to Keyword Intent Matching
Now, deciding what makes quality and relevant content is something Google has been focusing on for a while.
In 2013, Google revamped its main algorithm and named it Hummingbird.
Before this, Google’s scoring of whether a page was deemed relevant or not, was largely related to how well it had been keyword optimized.
If you include keywords into important on-page elements like title tags, headings and within the body of the content, you would score highly. You can think of this approach as relevancy 1.0.
However, one of Hummingbird’s main goals was to move away from “strings, to things” by better understanding the meaning of keywords and how they related to other topics, rather than the string of characters that make up a word.
Fast forward a couple of years (2015), and Google introduced Hummingbird’s first new sub-algorithm: RankBrain.
It was special because it was Google’s first artificial intelligence algorithm.
RankBrain better understands the user intent behind a keyword, and it focuses on providing the best result possible, rather than the best keyword optimized result.
Google has gone public in saying RankBrain is one of its top three rankings factors.
So, here’s what you need to know… there is now an increasing shift away from merely keyword matching a search query to satisfying the underlying keyword intent of a query.
If you think about it, it makes a lot of sense. Google now uses artificial intelligence in an attempt to work out when a user is satisfied or not. Keyword intent matching is fast becoming one of the most important aspects of modern SEO.
So, what’s the difference between BERT and RankBrain?
Well, let’s start with the similarities. Both are controlled by Google’s main algorithm Hummingbird, use artificial intelligence and focus on understanding the intent behind a search query, so they can help deliver the best result possible.
RankBrain excels on understanding the user intent for long-tail, more specific and unknown keywords; while BERT tackles the finer nuances of natural language.
When you start to think about the user intent behind a keyword and what the best results might be for the user, you have graduated your thinking from relevancy 1.0 (keyword matching) to relevancy 2.0 (keyword intent matching).